Long gone are the days when the fashion executive could set her product lines seasons in advance of a debut with shoppers. The trend towards individual expression fueled by social media combined with a couple of decades of priming by fast fashion brands has led us to a ravenous appetite for dynamism. The legacy seasonal cycle has been dismantled into shorter release sprints that see new capsule collections and product lines launched year-round.
All of this juxtaposed with demand for products that align with a cultural focus around important issues like sustainability and inclusiveness. The executive and merchant have their work cut out for them. This is no task for the faint-hearted. With this in mind, we got to work, building an algorithm-led solution that delivers ears for the internet so that trend and product line iterations can quickly incorporate granular intelligence from the frontline.
Our Digital Trend Foresights solution answers questions like the following, continuously and dynamically, as the marketplace shifts:
- What new trends should I onboard?
- How should I calibrate established trends to maximize my chances of success?
- Are the established trends increasing or decreasing in power?
- What trend themes and product lines do I send to manufacturing and ordering?
- What product lines to stock up on mid-cycle?
- How should I markdown and execute end-of-lifecycle planning across my portfolio?
- What should I discontinue to optimize inventory storage and warehousing?
- And more…
So how does it work?
There are four key steps that work together in a highly automated and scalable fashion! The outcome is a drastic increase in the confidence that an executive or merchant can have in his or her choices related to future product planning and assortment.
- Analysis of history
- Tracking of established and emerging product attributes
- Evaluation of the performance of key metrics
- Analysis of the probability of future success
1. Analysis of history
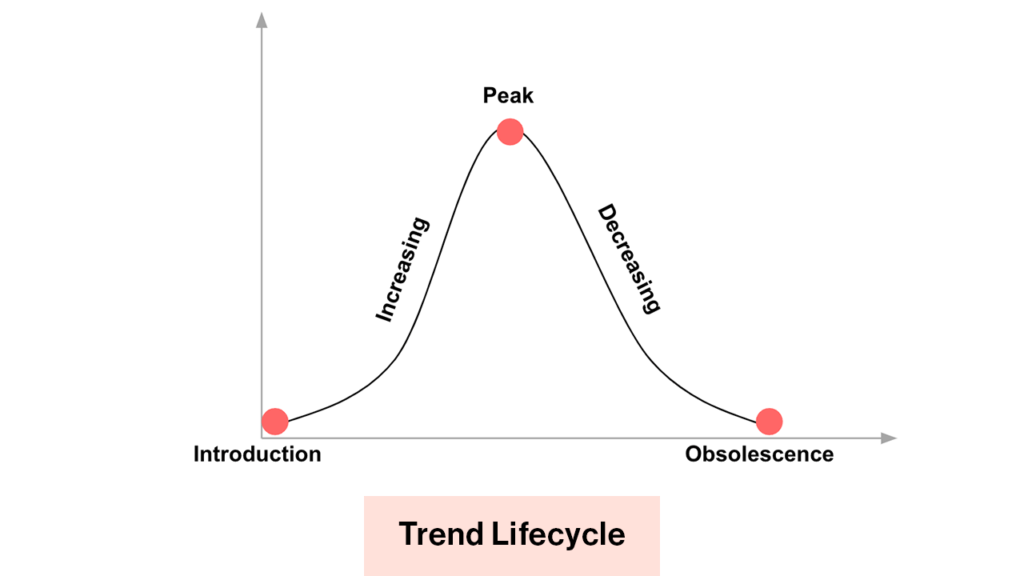
As our data science team says, the evolution of a trend or attribute (a style) can have multiple possible outcomes. This means that on the extreme ends of the scale certain product trends and attributes will make that product a hit or a flop with shoppers. Our machine pinpoints where a product is in its lifecycle, and how it is resonating with the shopper audience. This reveals insight into a product’s likelihood of future success. The machine can also gain insight into the likelihood of future success of similar products that are still in earlier and emerging phases of their lifecycle.
2. Tracking established and emerging product attributes
The algorithm is able to identify and understand themes and trends around a given product through analyzing unstructured data from the bottom up. This is important because it allows the algorithm to differentiate between exactly what is winning and what is losing in the marketplace.
Let’s take the example of recycled jeans.
First, the algorithm mines the product copy for keywords capturing recurring instances from the product name, description, and stated attributes across all relevant competitor websites of our clients. It uses our own comprehensive attribute libraries to marry and merge attribute synonyms that are informed by over one billion products! This is further enhanced with a cutting-edge technique called computer vision. Computer vision is a branch of Artificial Intelligence (AI) that enables our system to interpret and act on important information from visual inputs such as images and videos.
Secondly, we hone in on the keywords, such as “recycled and upcycled” that relate to the attribute of “recycled jeans”.
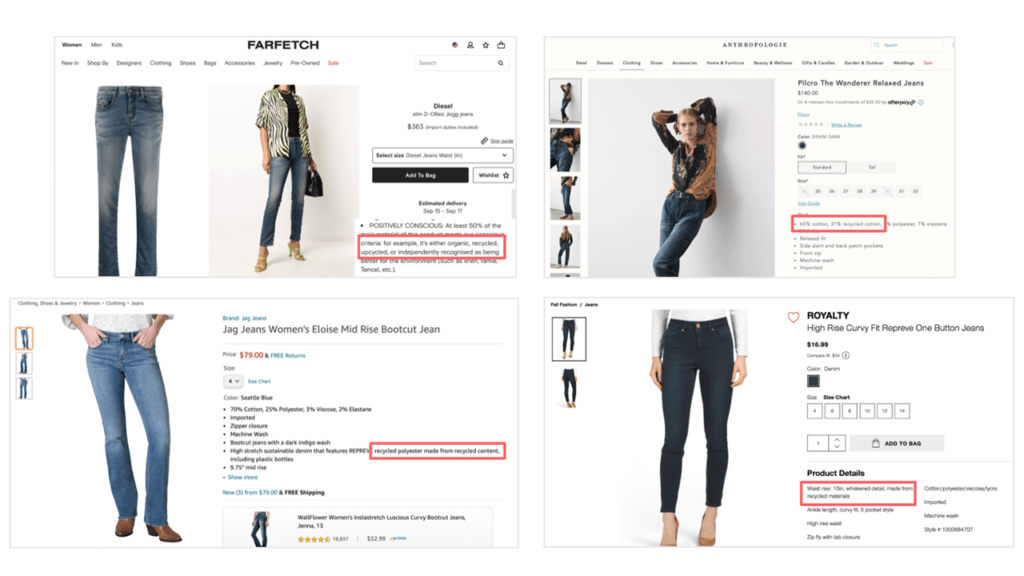
Our methodology then creates logical clusters of these keywords that derive a theme, in this case, environmental consciousness.
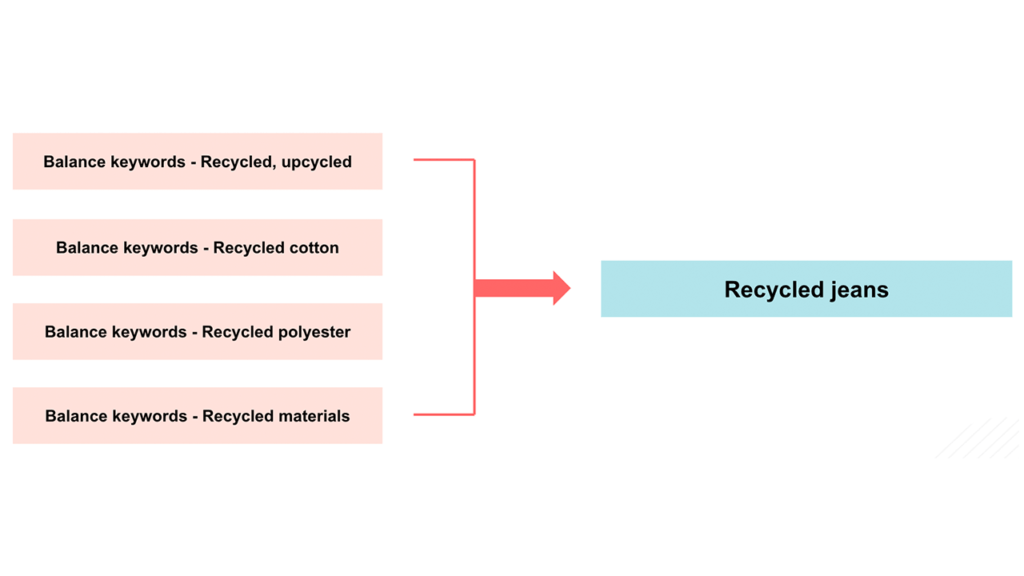
The model then goes one step further to look for context similarity. It looks for similar keywords such as “organic jeans” that revolve around the identified theme of environmental consciousness.
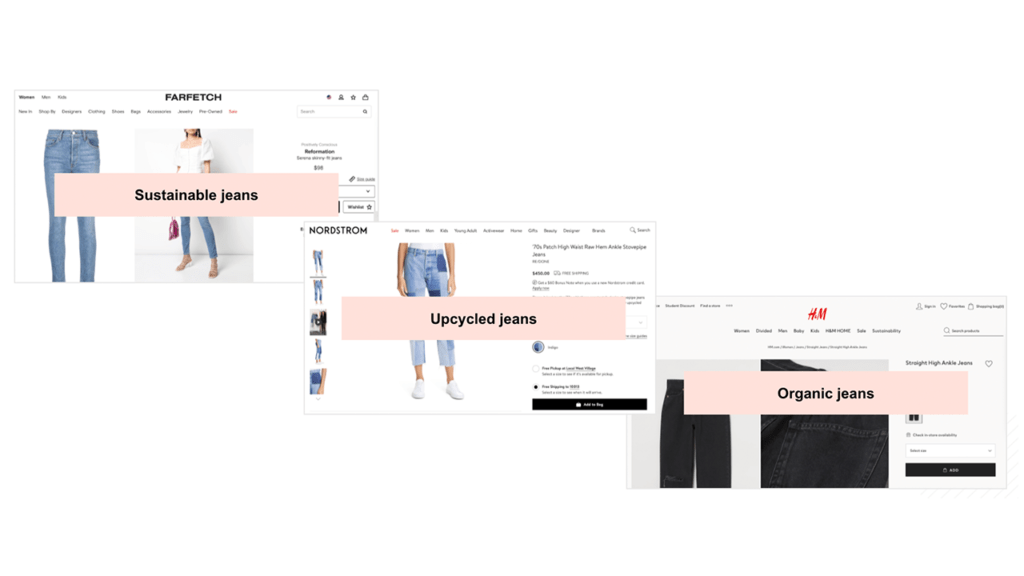
3. Evaluation of the performance of key metrics
Our machine analyzes metrics such as share of shelf, power rank and time rank to evaluate the performance of the identified trends, attributes and products to infer their probability of future success.
a. Share of digital shelf is the percentage visibility a product gets from a keyword or attribute inquiry, for example, “Mom Jeans”.
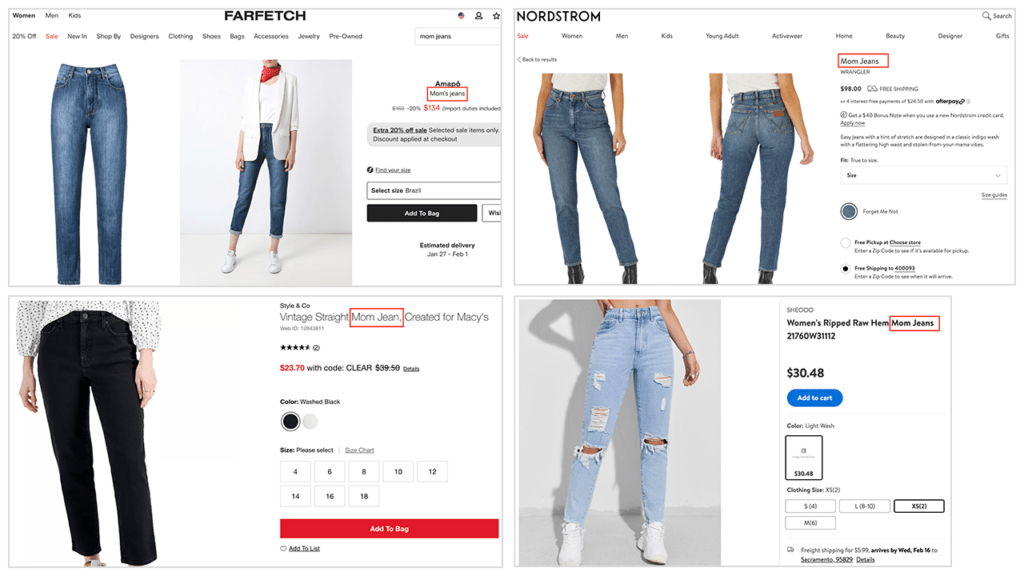
b. Power rank pulls in a joint score on KPIs related to shopper interest:
- Customer reaction: The number and velocity of product reviews and ratings. Our algorithm counts all customer reviews across websites along with the velocity of these reviews to analyze customer sentiment for an attribute such as “Mom Jeans”
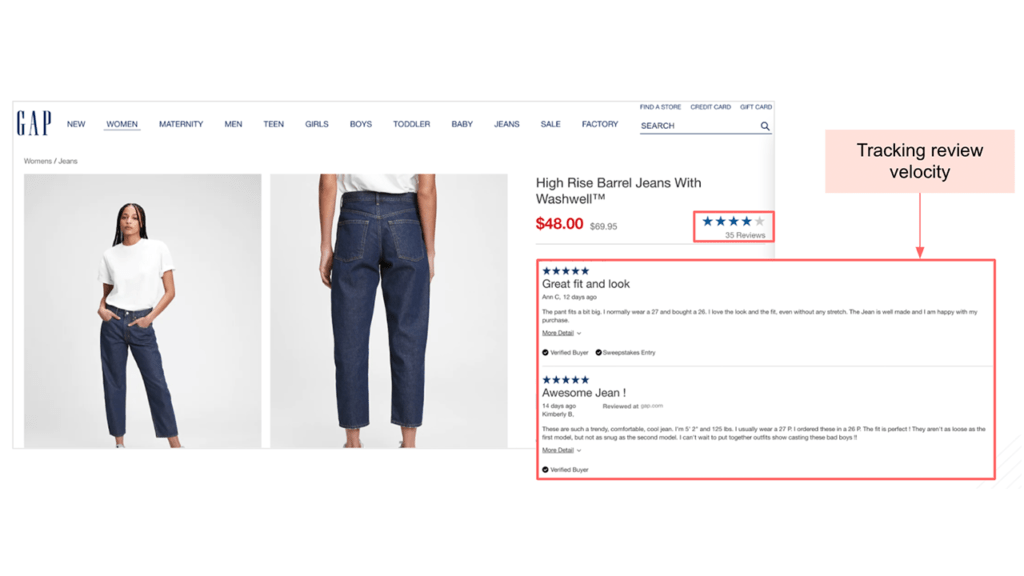
- Customer action: Tracking of sales velocity and product visibility across domains is done by continuously collecting inventory counts from multiple sources including the product page, shopping cart, the Amazon buy box, and more. The machine looks at the frequency at which the inventory levels are depleting over a time period. Faster depletion equates to greater sales velocity and higher resonance with shoppers.
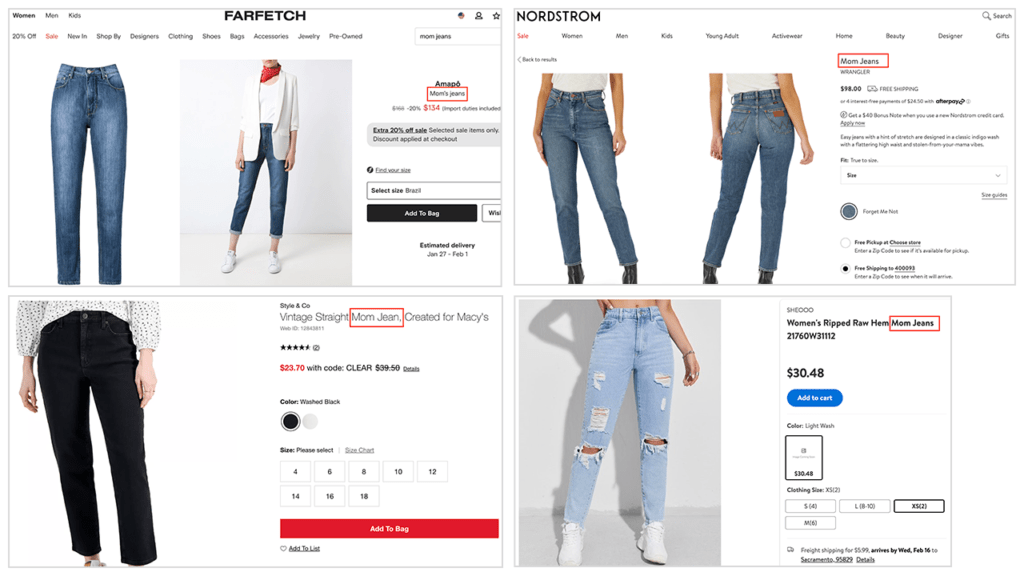
- Customer discoverability: Tracking product searchability and popularity by using the search algorithm of your competitor’s website. The algorithm tracks the first 100 products on competitor domains and analyzes the share of every attribute. The algorithm also tracks weekly and monthly popularity of attributes, such as “Mom Jeans” on Google trends. This ongoing search data is a direct reflection of interest in the trend, attribute or product across the defined regions.
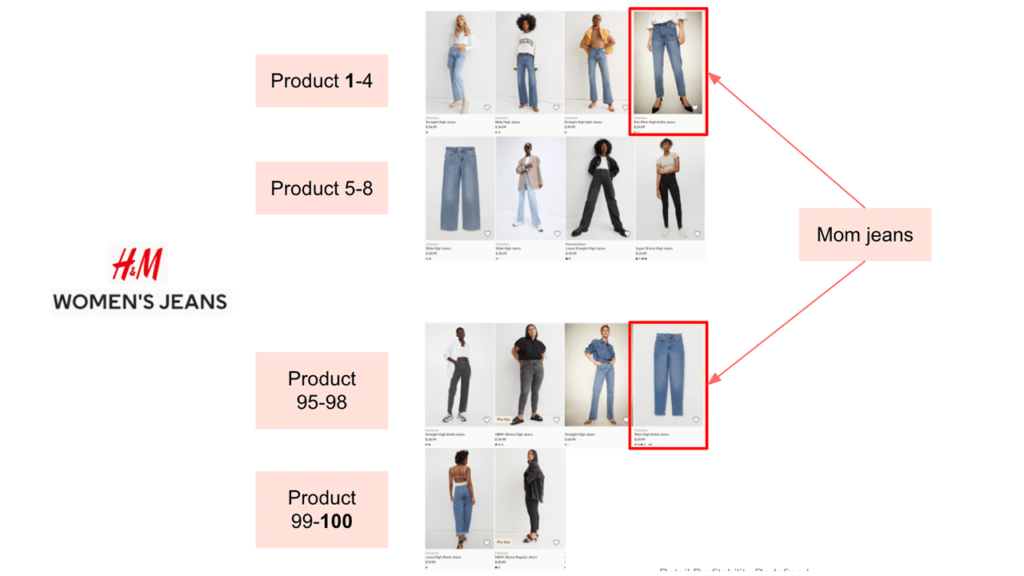
“Mom jeans” accounts for 26% of the first 100 products listed on H&M.
Also read : 8 Ways Retailers Can Leverage Customer Reviews Using Machine Learning
- Product Lifecycle: The algorithm analyzes product lifecycle dynamics by tracking key product activities at an attribute level. This includes things such as product promotions, discount ratio, product affinity, product availability, rate of replenishment, average span of product stock outs, and more.
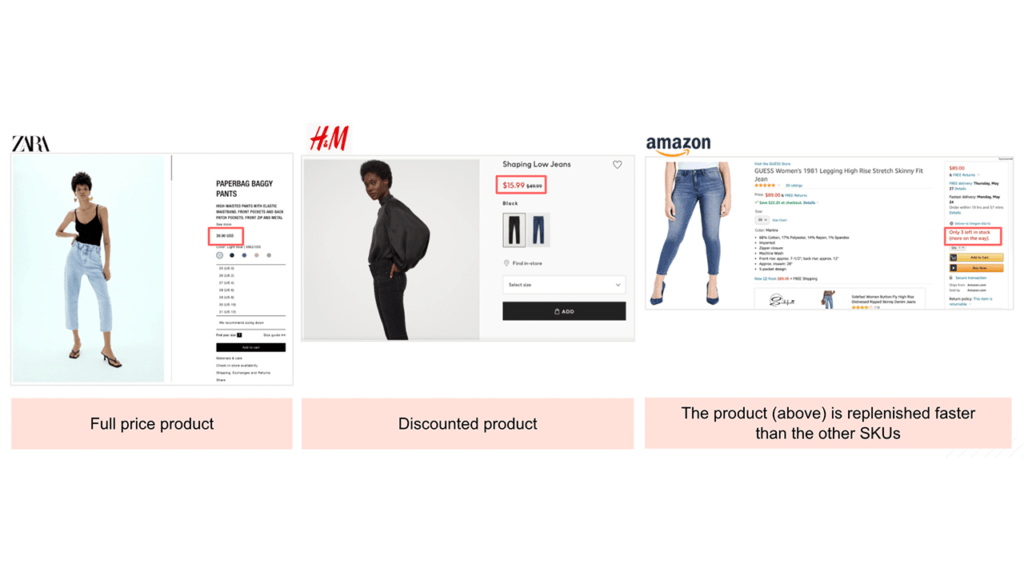
c. Time rank: This involves tracking the age of an attribute which helps to identify new trends at an early stage and also sheds light on declining trends across the marketplace.
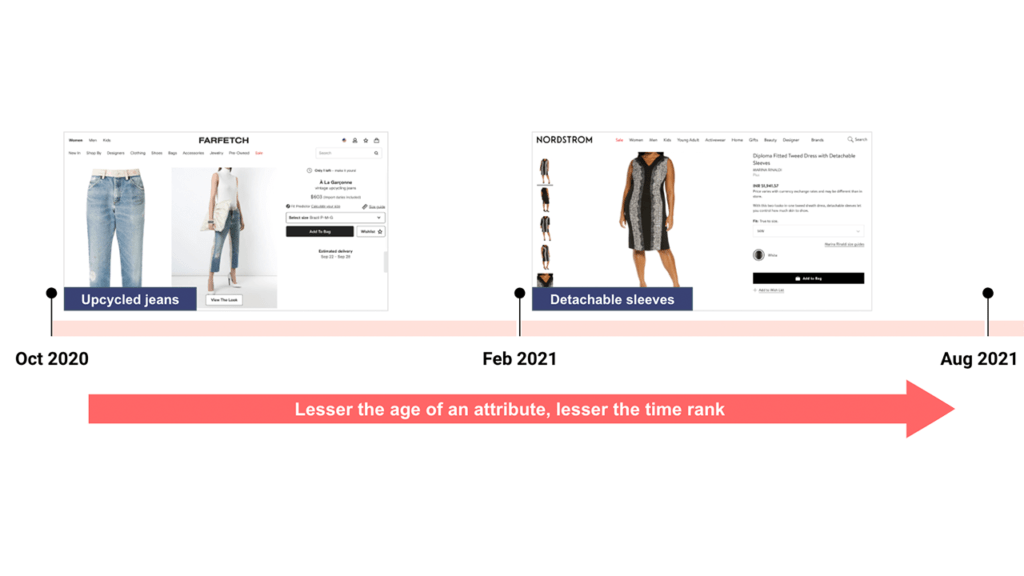
4. Analyzing the probability of future success
Based on the performance of the metrics covered in step 3, the machine then identifies which trends and attributes are likely to make a product a hit or a flop in the future.
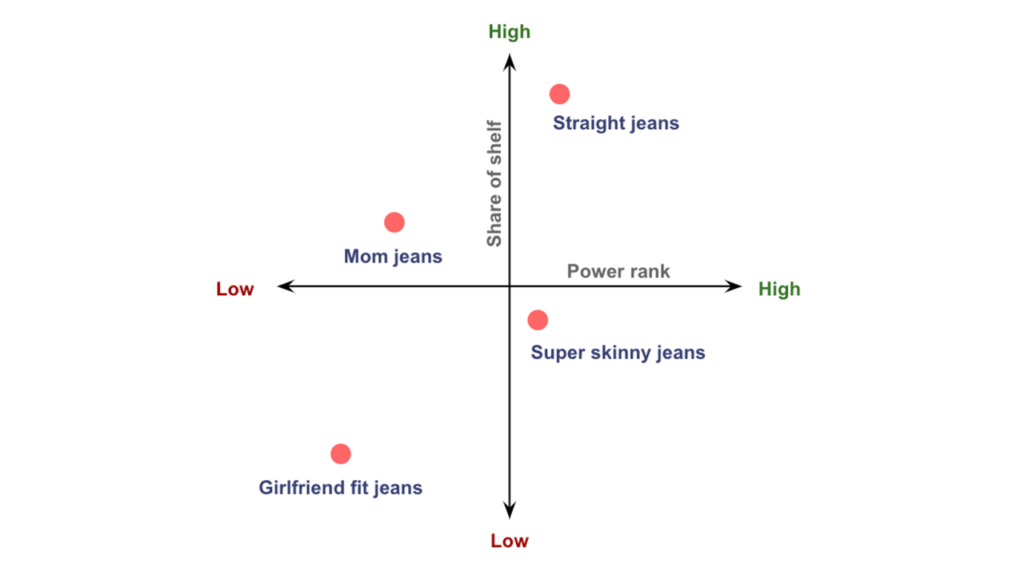
In the above example, we looked at the performance of key metrics for ‘straight fit jeans’ over the past 24 months. By applying this algorithm-led approach, we can predict the probability of success for straight fit jeans months into the future.
This methodology is a new market-driven approach to identifying future trends and informing product strategies that lead to greater resonance with shoppers, and in turn, more efficient and profitable outcomes for the brand and retailer. Executives and merchants gain a great deal of confidence in the trend and product decisions they are able to drive for their businesses. Furthermore, the solution is able to absorb a level of insight and analytics work that no team of hundreds of humans possibly could. This in turn frees up the executive to focus on the art of her go-to-market strategy that can then be married with the data to produce the most powerful business result outcome. Digital Trend Foresights complements any existing trend forecasting methodologies and practices that retailers and brands have in place with data that reflects real-time shopper behavior.
Final word
The pace at which shopper preferences are evolving today calls for brands and retailers that are able to harness the power of machine-driven data intelligence in their go-to-market strategies. Do art and intuition still matter? Of course they do. A business would be lost without them but no longer are they enough. Leveraging advanced analytics will help retailers derive insights that identify future trends and shopper preferences at an otherwise impossible level of precision and pace. This is exactly what Intelligence Node’s solution – Digital Trend Foresights – is delivering for the retail ecosystem. The solution is currently being put to work by brands and retailers in mid-market apparel & fashion categories.
About Intelligence Node
Intelligence Node provides the most competitive intelligence for digital commerce.
With more than 1 billion unique products mapped across 190,000 brands for more than 1,400 categories across 100+ languages every minute – this is the machine feeding the growth of more than $600 billion in retail revenue globally. It is the platform of choice for hundreds of retailers and brands worldwide, including Fortune 500 retailers and category leaders like Nestle, Prada, LIDL, SSENSE, Li & Fung, John Lewis, Lenovo and many others.
Led by a revolutionary team of experienced executives and leaders from fashion, retail, big data and eCommerce sectors, Intelligence Node is backed by top investors including MegaDelta Capital and Orios Venture Partners.